Beici Liang, Centre for Digital Music, Queen Mary University of London
Description:
Deep Pedal reveals the secrets of expressive piano playing through analysis and modelling of the pedalling gestures and techniques. Informed by piano acoustics and music theory, it elaborates on the studies in the sensor and audio domains, applying signal processing and deep learning techniques to better event detection.
Main Features:
– Measurement, recognition and visualisation of piano pedalling gestures and techniques
– Exploiting musically motivated audio features for legato-pedal onset detection
– Employing deep learning to identify sustain-pedal segments in piano music
– Facilitating the development of piano transcription and pedagogy applications
Main Publications:
Beici Liang, György Fazekas, Mark Sandler. “Measurement,Recognition and Visualisation of Piano Pedalling Gestures and Techniques”, Journal of the Audio Engineering Society Volume 66 Issue 6 pp. 448-456, 2018.
Beici Liang, György Fazekas, Mark Sandler. “Piano Legato-Pedal Onset Detection Based on a Sympathetic Resonance Measure”, in Proceedings of the 26th European Signal Processing Conference (EUSIPCO), 2018.
Beici Liang, György Fazekas, Mark Sandler. “Detection of Piano Pedalling Techniques on the Sustain Pedal”, Audio Engineering Society Convention 143, 2017.
Further Information:
Beici Liang (https://beiciliang.weebly.com/) is a PhD candidate at Centre for Digital Music and Media & Arts Technology Centre for Doctoral Training. Her research focuses on analysis and modelling of instrumental gestures.
Source code: https://github.com/beiciliang
Watch video demo of Piano Pedaller: https://youtu.be/nSjeV6R1S4k
Download Deep Pedal poster here: https://beiciliang.weebly.com/uploads/2/4/3/1/24316476/deeppedal-poster.pdf
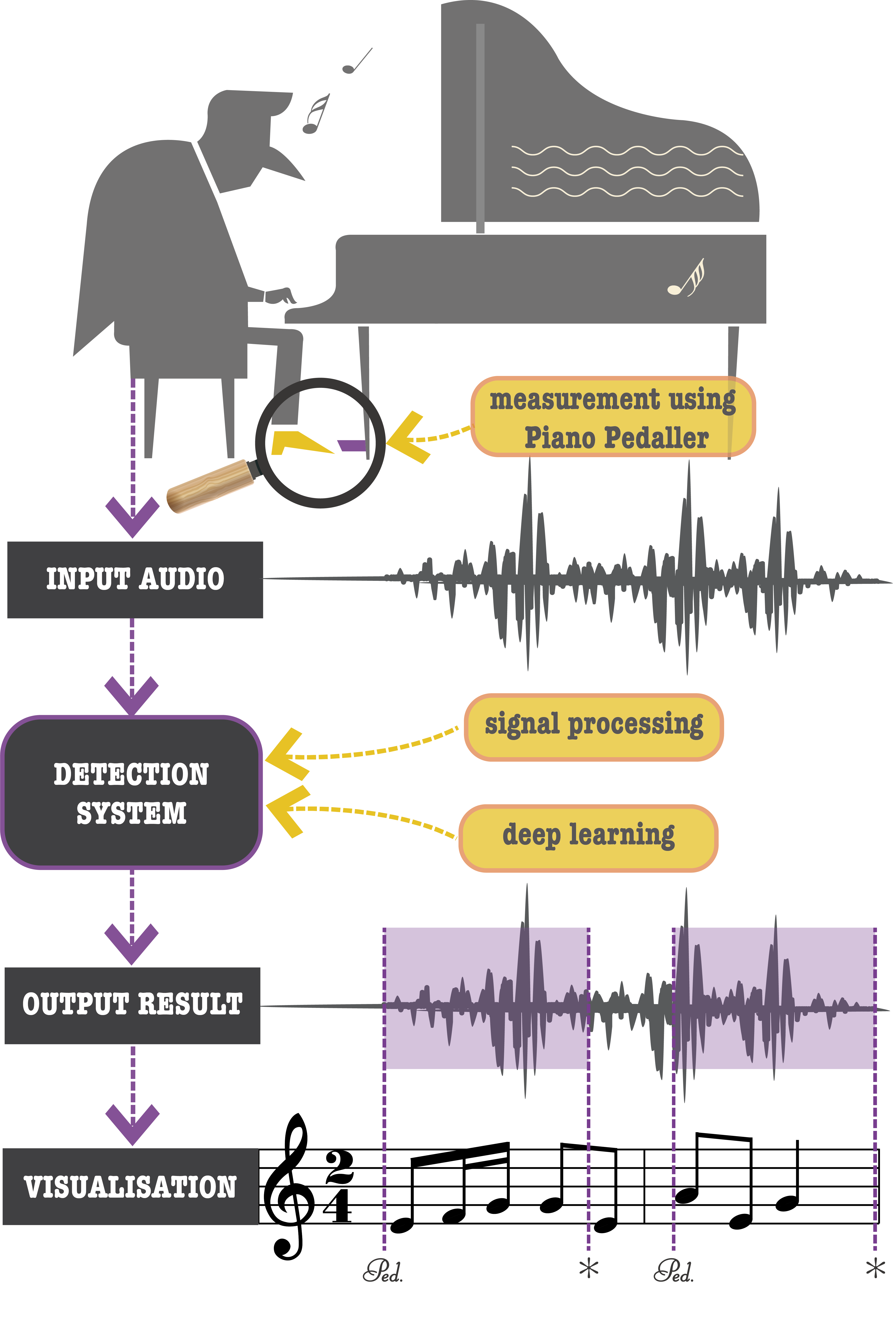