Delia Fano Yela, Centre for Digital Music, Queen Mary University of London
In recent years, source separation has been a central research topic in music signal processing, with applications in stereo-to-surround up-mixing, remixing tools for DJs or producers, instrument-wise equalising, karaoke systems, and pre-processing in music analysis tasks.
Sound source separation is part of a wider discipline aiming to extract the unknown individual sources from a given observable mixture of different sources. In our case, the sources are the ones typically found on a music recording set-up, such as musical instruments or audience noise.
Typically there are more sound sources than observable mixtures and very little or no information about the sonic environment of the recording, making it a very challenging task.
Different methods proposed in the past exploit a diverse variety of acoustical or perceptual aspects of the sources to help with their identification and separation.
The most recent trend employs Deep Neural Networks along side other AI techniques as they can achieve high audio quality results. However, one should be aware its performance heavily depends on the quantity and quality of the training data, which is also often not in the public domain.
The aim of this PhD is to explore and understand the diversity of these methodologies and their applications, to further identify their weaknesses and develop novel methods to improve the state-of-the-art and introduce new application scenarios. In particular, we focus on quick source separation methods that have the potential to be implemented in ‘real time’.
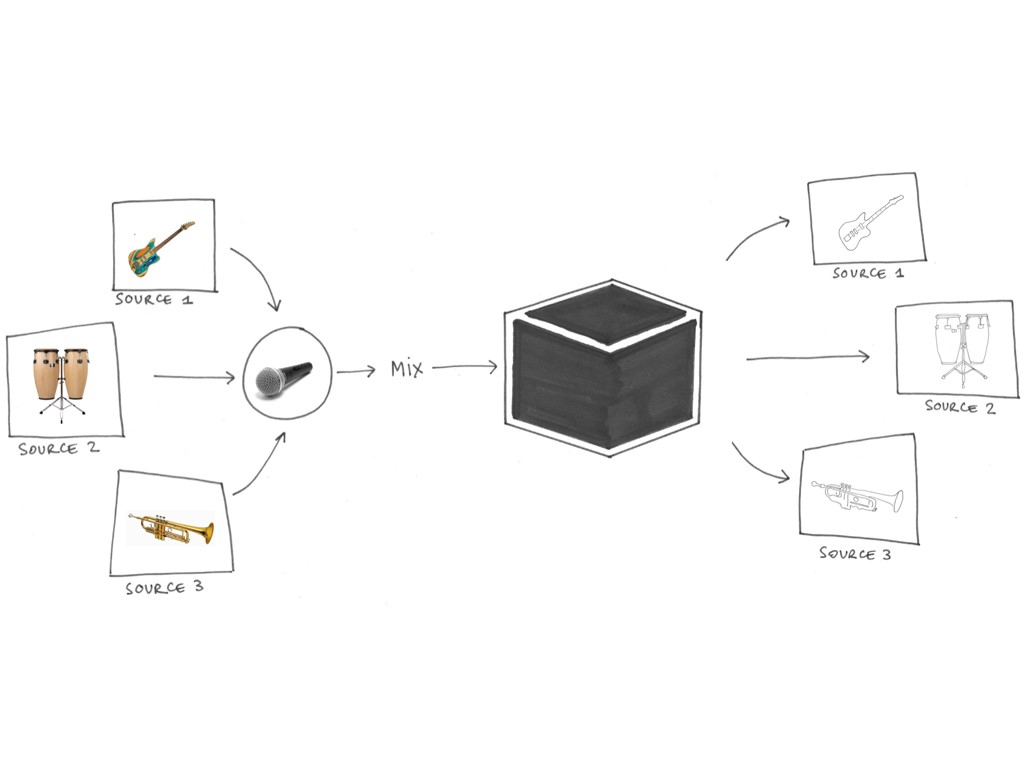