In August 2016, FAST interviewed Professor Meinard Muller, one of the partners on the FAST IMPACt project.
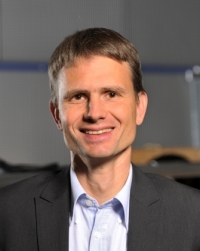
1. Could you please introduce yourself?
After studying mathematics and theoretical computer science at the University of Bonn, I moved to more applied research areas such as multimedia retrieval and signal processing. In particular, I have worked in audio processing, music information retrieval, and human motion analysis. These areas allow me to combine technical aspects from computer science and engineering with music – a beautiful and interdisciplinary domain. Since 2012, I hold a professorship at the International Audio Laboratories Erlangen (AudioLabs), which is a joint institution of the Friedrich-Alexander-Universität Erlangen-Nürnberg (FAU) and the Fraunhofer Institute for Integrated Circuits IIS. At the AudioLabs, I chair the group on Semantic Audio Processing with a focus on music processing and music information retrieval.
- What is your role/work within the project?
Within the FAST project, I see my main role as bringing advice and skills from music information retrieval and expertise in semantic audio processing. For example, in collaboration with researchers from the Centre for Digital Music (C4DM), we have developed methods for music content analysis (e.g. structure analysis, source separation, vibrato detection). Also, we have been working on the development of automated procedures for linking metadata (such as symbolic score or note information) and audio content. As we are conducting joint research, another important role is to support the exchange of young researchers between the project partners. We have had such an exchange in the last years by sending students and post-docs from the AudioLabs to the Centre for Digital Music, and vice versa.
- What, in your opinion, makes this research project different to other research projects in the same discipline?
One main goal of the FAST project is to consider the entire chain from music production to music consumption. For example, to support automated methods for content analysis, one may exploit intermediate audio sources such as multitrack recordings or additional metadata that is generated in the production cycle. This often leads to substantial improvements in the results achievable by automated analysis methods. Considering the entire music processing pipeline makes the FAST project very special within our discipline.
- What are the research questions you find most inspiring within your area of study / field of work?
Personally, I am very interested in processing audio and music signals with regard to semantically or musically relevant patterns. Such patterns may relate to the rhythm, the tempo, or the beat of music. Or one may aim at finding and understanding harmonic or melodic patterns, certain motives, themes, or loops. Other patterns may relate to a certain timbre, instrumentation, or playing style (involving, for example, vibrato or certain ornaments). Obviously, music is extremely versatile and rich. As a result, musical objects (for example, two music recordings), although similar from a structural or semantic point of view, may reveal significant differences. Understanding these differences and identifying semantic relations despite these differences by means of automated methods are what I find inspiring and challenging research issues. These issues can be studied within music processing, but their relevance goes far beyond the music scenario.
- What can academic research in music bring to society?
Music is a vital part of nearly every person’s life on this planet. Furthermore, musical creations and performances are amongst the most complex cultural artifacts we have as a society. Academic research in music can help us to preserve and to make our musical heritage more accessible. In particular, due to the digital revolution in music distribution and storage, we need the help of automated methods to manage, browse, and understand musical content in all its different facets.
- Please tell me why do you find valuable/ exciting / inspiring to do academic research related to music.
As already mentioned before, music is an outstanding example for studying general principles that apply to a wide range of multimedia data. First, music is a content type with many different representations, including audio recordings, symbolic scores, video material as provided by YouTube, and vast amounts of music-related metadata. Furthermore, music is rich in content and form, comprising different genres and styles – from simple, unaccompanied folk songs, to popular and jazz music, to symphonies for full orchestras. There are many different musical aspects to be considered such as rhythm, melody, harmony, timbre – just to name a few. And finally, there is also the emotional dimension of music. All these different aspects make the processing of music-related data exciting.
- What are you working on at the moment?
My recent research interests include music processing, music information retrieval, and audio signal processing. In the last years, I have been working on various questions related to computer-assisted audio segmentation, structure analysis, music analysis, and audio source separation. In my research, I am interested in developing general strategies that exploit additional information such as sheet music or genre-specific knowledge. We develop and test the relevance of our strategies within particular case studies in collaboration with music experts. For example, at the moment, we are involved in projects that deal with the harmonic analysis of Wagner’s operas, the retrieval of Jazz solos, the decomposition of electronic dance music, and separation of Georgian chant music. By considering different application scenarios, we study how general signal processing and pattern matching methods can be adapted to cope with the wide range of signal characteristics encountered in music.
- Which is the area of your practice you enjoy the most?
Besides my work in music processing, I very much enjoy the combination of doing research and teaching. I am convinced that music processing serves as a beautiful and instructive application scenario for teaching general concepts on data representations and algorithms. In my experience as a lecturer in computer science and engineering, starting a lecture with music processing applications – in particularly playing music to students opens them up and raises their interest. This makes it much easier to get the students engaged with the mathematical theory and technical details. Mixing theory and practice by immediately applying algorithms to concrete music processing tasks helps to develop the necessary intuition behind the abstract concepts and awakens the student’s fascination for the topic. My enthusiasm in research and teaching has also resulted in a recent textbook titled “Fundamentals of Music Processing” (Springer, 2015, www.music-processing.de), which also reflects some of my research interests.
- What is it that inspires you?
Because of the diversity and richness of music, music processing and music information retrieval are interdisciplinary research areas which are related to various disciplines including signal processing, information retrieval, machine learning, multimedia engineering, library science, musicology, and digital humanities. Bringing together researchers and students from a multitude of different fields is what makes our community so special. Working together with colleagues and students who love what they do (in particular, we all love data we are dealing with) is what inspires me.
Contact Details:
Prof. Dr. Meinard Müller
Lehrstuhl für Semantische Audiosignalverarbeitung
International Audio Laboratories Erlangen
Friedrich-Alexander Universität Erlangen-Nürnberg
Am Wolfsmantel 33
91058 Erlangen, Germany
Email: meinard.mueller@audiolabs-erlangen.de
Reference:
Müller, Meinard, Fundamentals of Music Processing, Audio, Analysis, Algorithms, Applications, 483 p., 249 illus., 30 illus. in color, hardcover, ISBN: 978-3-319-21944-8, Springer 2015. www.music-processing.de